Artificial intelligence in insurance: what are the risks?
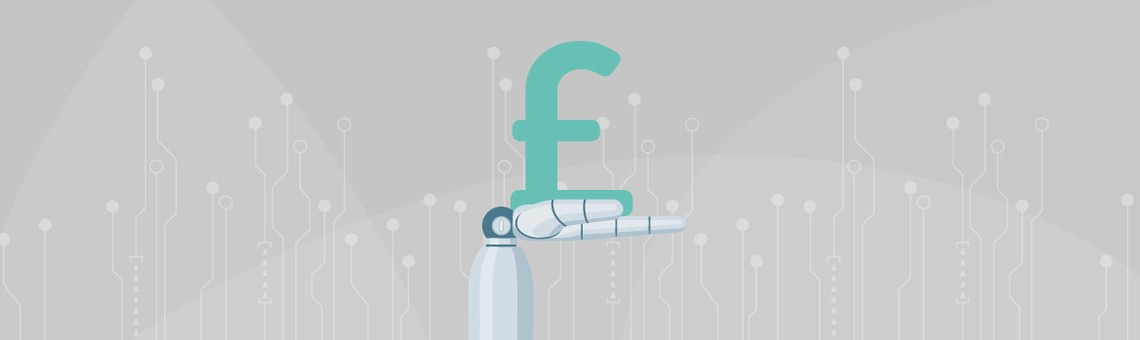
Estimated reading time: 4 minutes
The use of artificial intelligence (AI) within the insurance industry has been widely anticipated for years. Today this anticipation is reality.
Examples of this use of AI include Ageas and Tractable which, following a pilot in the fall of 2016, deployed an AI process that visually assesses car damage to provide estimates and generate approvals for repairs, thereby containing costs on Ageas’ motor insurance book. In other areas, the entry of InsureTech firms have seen the advent of the 60-second quote and the 3-second claim pay out, based on AI driven decisions.
While AI is gaining traction in the insurance industry, we should stop and ask the question of the risks that it presents from an accuracy, practical, legal or even ethical perspective. Below are just some of the issues to consider.
AI relies heavily on data. One source of data is the scraping of publically available information on the internet. This is a practice that has been long used in the industry. Whilst not in itself necessarily illegal, the data (factual or otherwise) may be subject to copyright. Using it without the copyright owner's permission, especially if you are selling it, could result in legal action. The ethics of data scrapping is a hot topic and we only have to think of the Facebook–Cambridge Analytica data scandal to see the potential for misuse. It was only a few years ago that Facebook forced a major UK insurer to abandon the scraping of Facebook public profiles for their risk selection process. Also, earlier this year Facebook sued Rankwave for a similar reason.
The use of such information would also need to be considered in the context of the General Data Protection Act (GDPR). Increasingly, GDPR notices are becoming more tick box exercises like the reading of terms and conditions. There may be issues concerning whether such consent is informed or freely given, depending on how the consent was presented.
AI systems are being used to support complex underwriting decisions. Underwriters should not make insurance eligibility decisions based on age, gender, race, religion or sexual orientation. However if not careful, an AI solution could make, or be designed to make, discriminatory eligibility decisions using hidden biases which are illegal and unethical. An AI system will only be as good as the data it uses.
For example, Amazon tested a recruitment AI tool, trained on data over a ten year period. The AI tool concluded that male candidates were preferable to female candidates based on the training data. For example, it looked for words like “women’s” and rated those CVs lower. It turns out that the applicant pool consisted of a higher proportion of male applicants as was common for that industry and the AI picked up on this unconscious bias.
One fear in the industry is that AI will lessen the need for human support, leading to increased potential for job losses in the insurance industry. Staff may have to be re-trained once the AI has settled in and insurers would need to plan for where to redeploy staff and understand what skills they require. However, in an increasingly cost conscious world, it may well be that these job losses become permanent. Either way, the disruption needs to be planned and handled carefully.
The other factor here is also complacency. Relying on AI to do the job could cause the human element to “down-skill." The 2018 fatality involving an Uber self-driving car was partly due to the failure of the backup driver to prevent the crash as she had become complacent due to the accuracy of the technology.
Insurance exists to pool risk. Increasingly, however, insurers are using AI to improve risk selection to drive through a more accurate price. However, this tends to go against the concept of pooling, which may lead to some segments of the population becoming uninsured. A simple example of this is attempting to insure houses on a flood plain - which in general would have been unaffordable had it not been for Flood Re.
Automated claims could lead to companies not being able to explain the decision making process if challenged by the claimant. The lack of transparency may also weaken the credibility of an insurer, even if the decision was the right one. Insurers will need to prepare for this in respect of the degree and complexity of response required, as well as skill and knowledge needed by their staff when responding.
A lack of transparency can also affect the operations of a business, thereby generating claims. The recent crashes of the Boeing 737 Max planes is an example of this, whereby pilot judgement was overridden by the on-board computer despite the computer being in error. However, the pilots were unable to react in a timely manner as they did not understand why the computer was behaving in the way that it did. The risk from such claims would worsen if extended to the world of automated vehicles.
The future of AI in insurance seems here to stay. However, we need to remember that AI (and the use of the data) will only be as good, bad or deviant as the society or companies that create and manage it.
Melanie Westcott contributed to the writing of this post.